Over the last year, artificial intelligence has moved swiftly from the realm of research into widespread practical applications. One institution that has taken notable steps to leverage AI technologies responsibly is the University of Michigan (U-M). Rather than relying entirely on commercial AI platforms, the university decided to develop its own closed generative AI tools. This initiative reflects not only strategic foresight but also a strong commitment to ethics, privacy, and academic integrity.
Known for its forward-thinking approach to technology in education, the University of Michigan saw the need to explore generative AI not as a consumer but as a builder. The move is not just about innovation—it’s about maintaining control over sensitive data and aligning AI development with the core values of higher education.
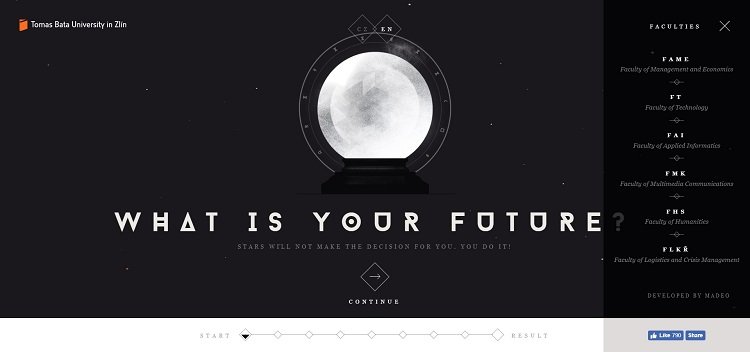
Why Build AI Tools In-House?
The decision to develop AI tools internally stems from several reasons rooted in the university’s mission and operational model. Here are the key motivations:
- Data Privacy and Security: Commercial AI tools often depend on cloud-based infrastructure that transfers user input and interactions to external servers. For a university that handles vast amounts of student and faculty data, this raised immediate privacy red flags. Building AI solutions in-house allows the university to limit data exposure and maintain control.
- Academic Integrity: U-M is particularly cautious about how AI could influence learning and assessment. By creating custom AI tools, educators have the opportunity to embed academic standards directly into the software architecture, minimizing misuse in classroom settings.
- Customization and Relevance: Off-the-shelf generative AI models may not align with specific academic use cases. Developing proprietary tools allows the university to tailor the AI’s outputs to research, teaching, and administrative needs without depending on generic solutions.
How the University Designed Its Internal AI
To build these tools, the University of Michigan assembled a multidisciplinary team that included computer scientists, ethicists, and instructional designers. The focus was not just on capability but also on values such as transparency, inclusivity, and accessibility. The project, housed under U-M’s Information and Technology Services (ITS) and the Center for Academic Innovation, took a modular approach:
- Foundational Models: Rather than build models entirely from scratch, U-M leveraged open-source transformer models and fine-tuned them on curated and ethically sourced academic content.
- Private Infrastructure: The models run on servers hosted within university-owned data centers, ensuring limited external access and aligning with FERPA (Family Educational Rights and Privacy Act) compliance standards.
- User-Centric Interfaces: Tools were integrated into existing platforms like Canvas and MWireless, designed with both student and faculty feedback to ensure intuitive adoption.
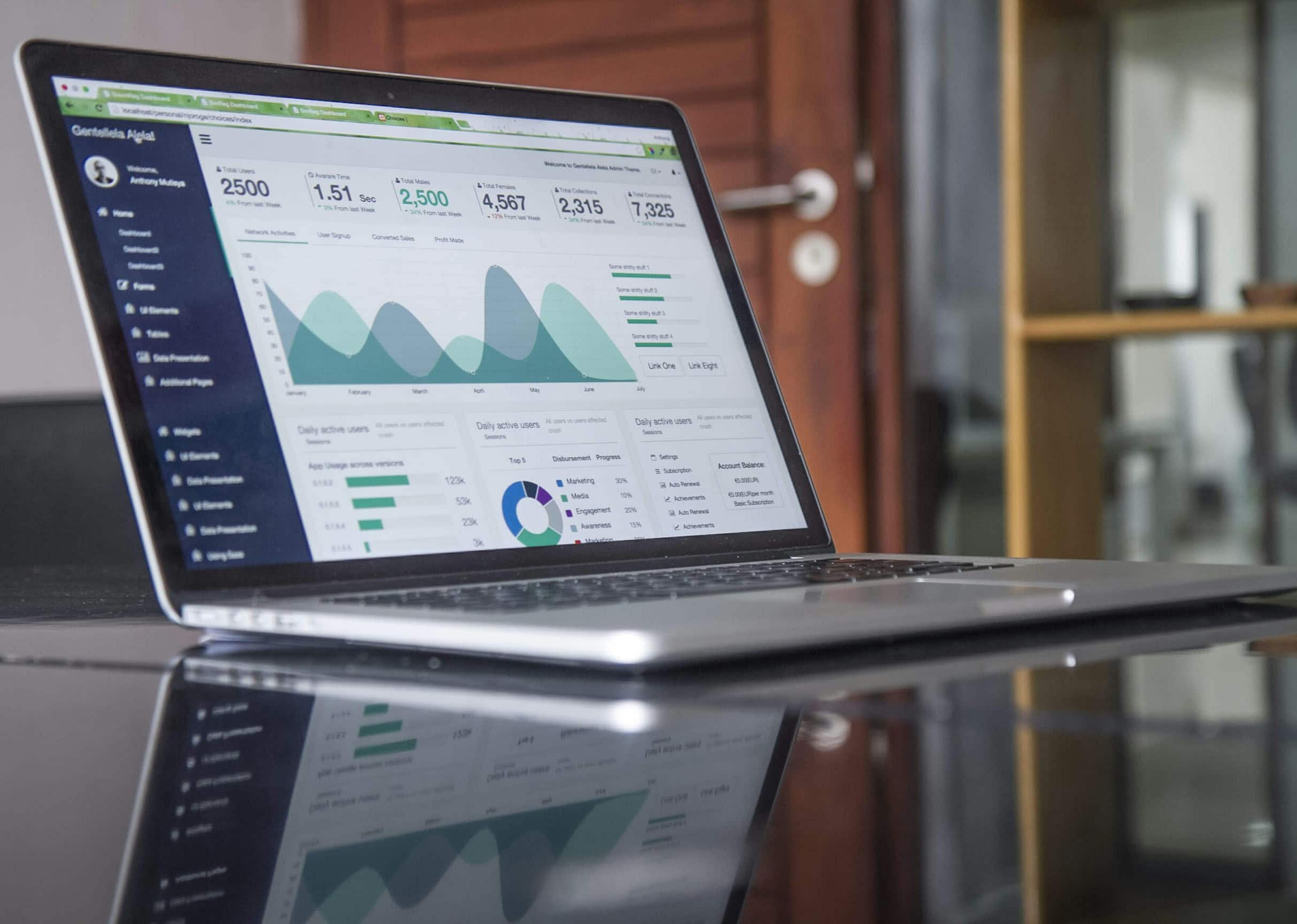
Early Use Cases and Adoption
The first wave of generative AI tools at U-M includes:
- AI-enhanced writing assistants incorporated into digital writing centers that provide feedback on clarity, style, and tone—without overstepping into authorship or generative text completion.
- Lecture summarization tools that convert long-form lectures into digestible bullet points and key insights, helping students with diverse learning needs.
- Faculty research assistants capable of parsing large academic databases and generating brief synthesis reports to support early research drafting processes.
These tools are rolled out gradually across different departments to assess their benefits and limitations. The university monitors usage closely, ensuring consistent alignment with pedagogical goals and student success frameworks.
Ethics and Oversight
One of the core challenges of implementing generative AI in educational institutions is maintaining strong ethical oversight. At U-M, this is being managed by a dedicated ethics review board composed of faculty across various disciplines. This board evaluates AI tools on an ongoing basis to ensure they:
- Provide equitable access to all students regardless of discipline or background
- Do not propagate bias or misinformation
- Comply with legal and academic standards
Transparency reports published bi-annually keep the university community informed about what tools exist, how they work, and what data is being used. This approach is aimed at fostering trust while preventing algorithmic opacity—a common concern in commercial AI systems.
Looking Forward
The University of Michigan’s path toward proprietary generative AI tools offers a potential model for other institutions seeking to balance innovation with responsibility. Instead of being dictated to by technology trends, U-M is shaping AI development based on its unique academic mission and the values it upholds.
As higher education continues to explore AI’s capabilities, Michigan’s self-developed ecosystem of tools may prove to be a bellwether in a growing trend toward institutional AI sovereignty—where academia reclaims control over the technologies that increasingly influence teaching, learning, and research.